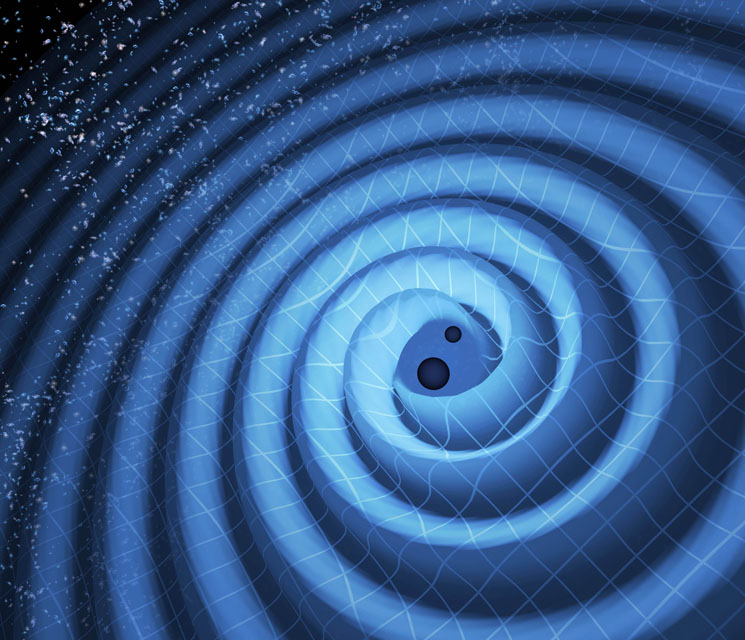
WG1Machine Learning for Gravitational Wave astronomy
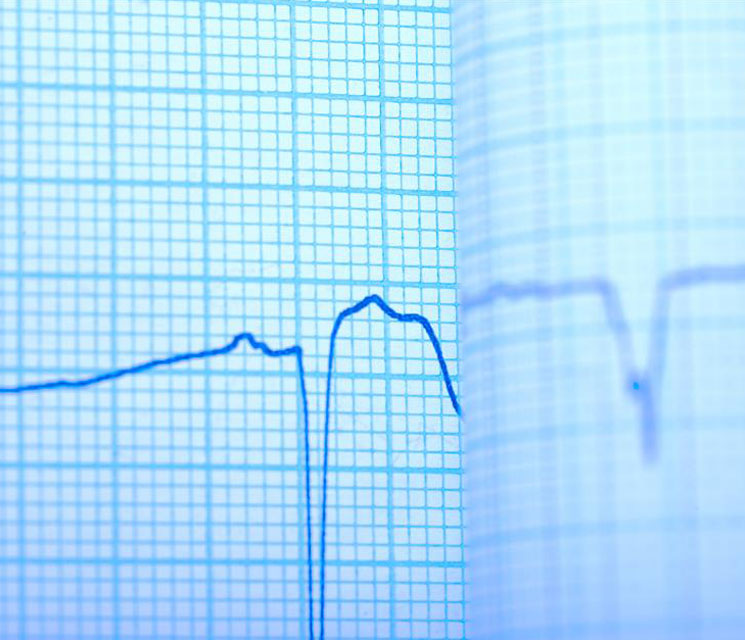
WG2Machine Learning for low-frequency seismic measurement
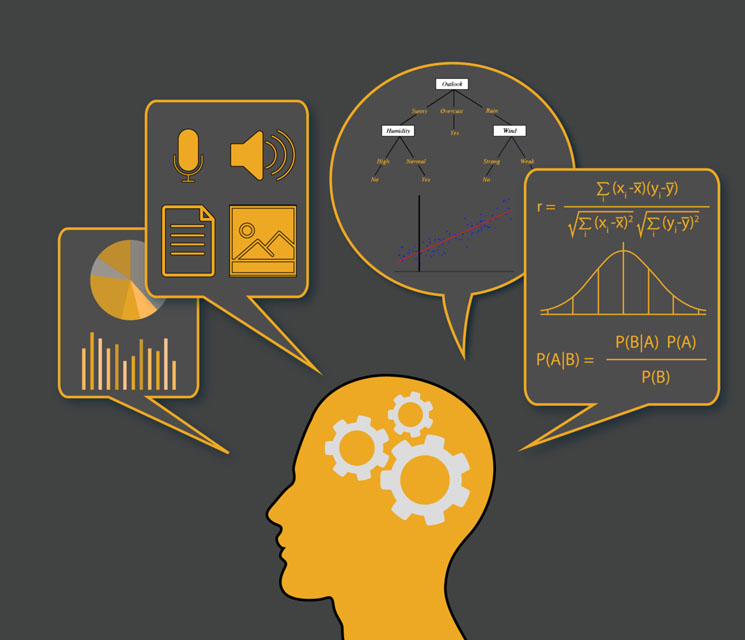
WG3Machine Learning for Advanced Control techniques
JOIN THE ACTION
The inclusion of further partners from currently participating COST Countries, or other countries within or outside the COST Network, is welcome and strongly encouraged during the entire duration of the Action.